Project 2: Email Spams Frequency Classification
- Hoanglan Nguyen
- Jan 11, 2022
- 2 min read
Updated: Feb 26, 2022
Introduction
Email is the primary communication tool for both business and personal use. We use emails in (almost) our daily life since they are very convenient to transfer text, program files, spreadsheets, and even photographic images. There are times when email spams exist within your email dashboard and you may want to get rid of but you weren't sure whether they are spam or not. During this project, I will use the classification algorithms such as
KNN and Naïve Bayes to determine the particular word or character that was frequently occurring in the e-mail.
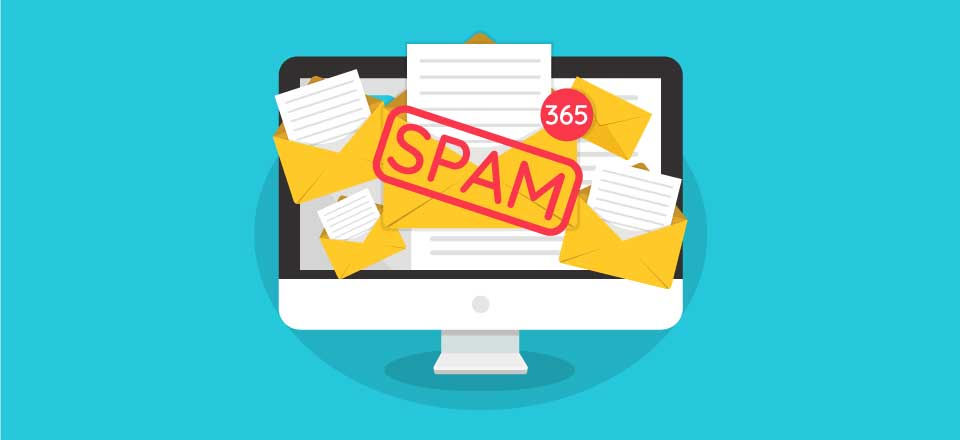
Introduction to the Dataset
The dataset I will be using the spam email classification created by user Somesh Sharma which contains several attributes that determines whether the email is considered spam or not spam i.e. unsolicited commercial e-mail. In this dataset, the collection of non-spam e-mails came from filed work and personal e-mails, and the word 'george' and the area code '650' are indicators of non-spam. Somesh Sharma call these useful when constructing a personalized spam filter.
Most of the attributes represents the words that makes frequent appearance on the emails. Some of the attributes may be based on characters, capital letters' length, the number of capital letters, the length of uninterrupted sequences of capital letters, and whether the e-mail was considered spam.
There are 48 wordfreqWord attributes:
Word_freq_make
Word_freq_address
Word_freq_all
Word_freq_3d
Word_freq_our
Word_freq_over
Word_freq_remove
Word_freq_internet
Word_freq_order
Word_freq_mail
Word_freq_receive
Word_freq_will
Word_freq_people
Word_freq_report
Word_freq_addresses
Word_freq_free
Word_freq_business
Word_freq_email
Word_freq_you
Word_freq_credit
Word_freq_your
Word_freq_font
Word_freq_000
Word_freq_money
Word_freq_hp
Word_freq_hpl
Word_freq_george
Word_freq_650
Word_freq_lab
Word_freq_labs
Word_freq_telnet
Word_freq_857
Word_freq_data
Word_freq_415
Word_freq_85
Word_freq_technology
Word_freq_1999
Word_freq_parts
Word_freq_pm
Word_freq_direct
Word_freq_cs
Word_freq_meeting
Word_freq_original
Word_freq_project
Word_freq_edu
Word_freq_table
Word_freq_conference
The percentage of words in the e-mail that match WORD, i.e. 100 * (number of times the WORD appears in the e-mail) / total number of words in e-mail. A “word” in this case is any string of alphanumeric characters bounded by non-alphanumeric characters or end-of-string.
I will take the first 10 words of this list so that way it makes easier to analyze rather than just have all 48 words. The new table is displayed in "preprocessing" section.
There are more attributes:
6 continuous real attributes of charfreqCHAR = percentage of characters in the e-mail that match CHAR, i.e. 100 * (number of CHAR occurrences) / total characters in e-mail
1 continuous real attribute type capitalrunlength_average = length of uninterrupted sequences of capital letters.
1 continuous integer attribute of type capitalrunlength_total = length of longest uninterrupted sequence of capital letters.
1 continuous integer attribute of type capitalrunlength_longest = sum of length of uninterrupted sequences of capital letters = total number of capital letters in the e-mail.
1 nominal class attribute of type spam = denotes whether the e-mail was considered spam (1) or not (0), i.e.
In summary, the words and characters are seen frequently within the emails and have to identify it whether it is a spam or not a spam.
Preprocessing the Data
During the preprocessing phase, I created a new notebook file and named it as project 2. Then, I imported the dataset csv file for me to analyze.

This is isn't the full table of this dataset but this show that I successfully imported the csv file into JupyterLab.

The new table that I created for the first 10 words that are seen frequently in any emails:

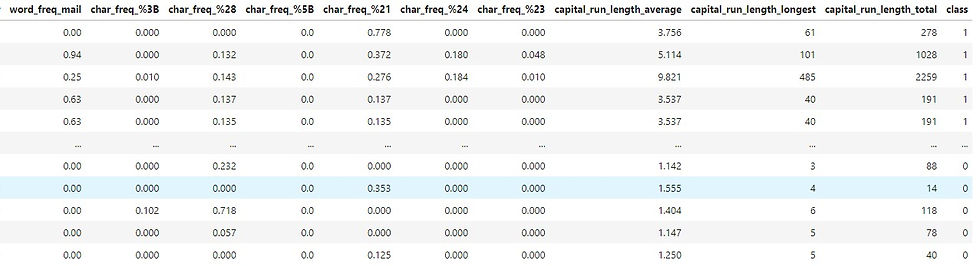
Data Understanding
Modeling
WIP
Evaluation
WIP
Storytelling
WIP
References
Codes
WIP
Comments